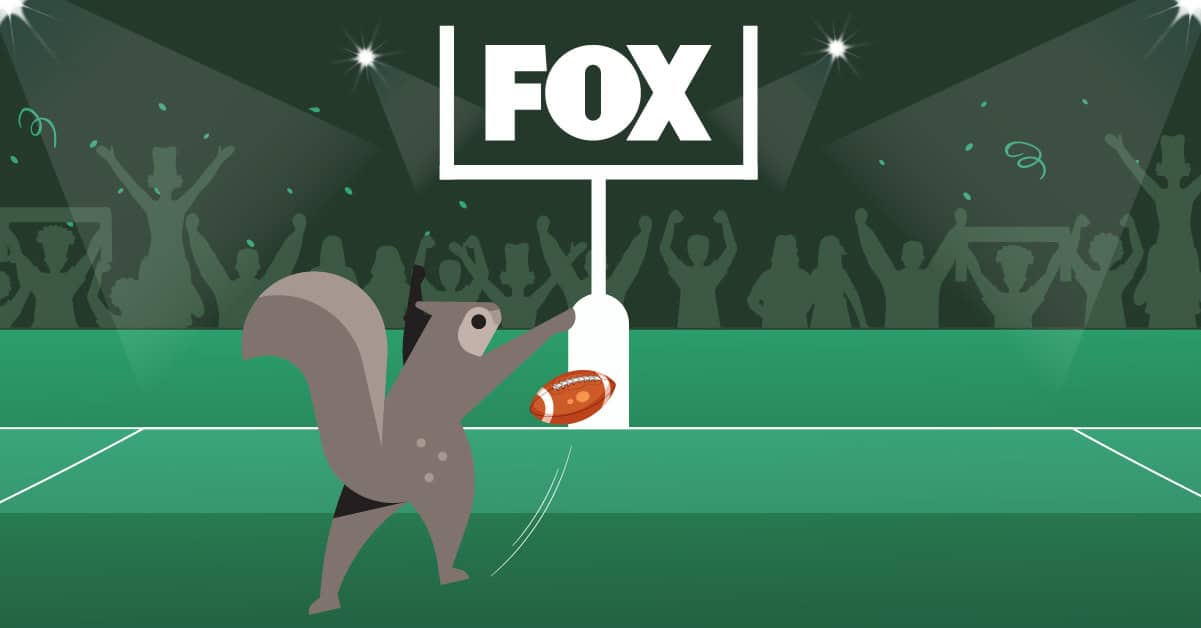
FOX Monitors Super Bowl Viewership Experience with Real-time Data Insights
FOX’s Media Collection and Viewership Beaconing Service (MCVBS) –a system designed to gather and analyze viewer data–powered by Momento, Amazon Athena, and Amazon Simple Storage Service (Amazon S3), delivered uninterrupted real-time metrics and accurate post game insights during the record-breaking Super Bowl LIX.